I'm a Doctoral Researcher at the Computational Social Science (CSS) Department at GESIS - Leibniz Institute for the Social Sciences. I'm fortunate to be advised by Prof. Claudia Wagner. My work is located at the intersection of NLP and social science. I am particularly interested in solving problems in hate and abuse speech on online social media platforms. My research objectives and interests are outlined here.
Before GESIS, I was a research intern in Prof. Rajesh Sharma‘s Computational Social Science Group. If you'd like to chat with me, feel free to schedule a meeting through my Google Calendar appointment link
Click for a surprise! 🤗
News
- Apr 2024 New Pre-print - "Analyzing Toxicity in Deep Conversations: A Reddit Case Study"
- Feb 2024 Our paper got accepted to the 2024 Joint International Conference on Computational Linguistics, Language Resources and Evaluation (LREC-COLING 2024)
- Feb 2024 I will be joining the Computational Social Science department at GESIS starting in March 2024
- Aug 2023 Our paper got accepted to the 32nd ACM International Conference on Information and Knowledge Management (CIKM 2023)
Publications
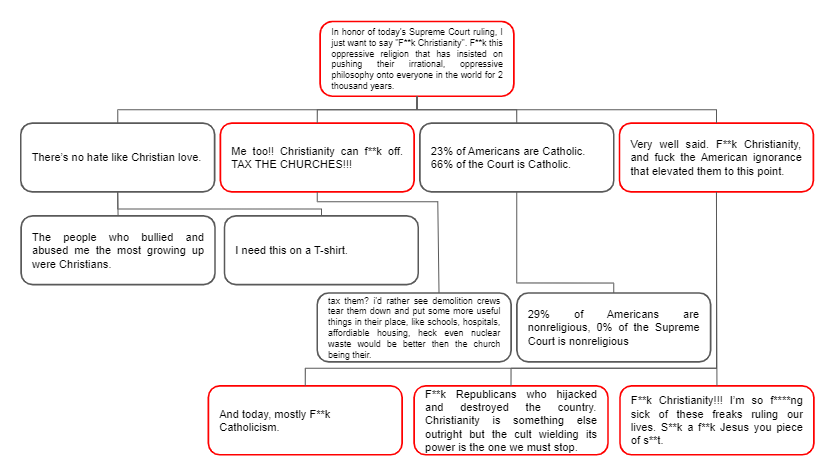
Analyzing Toxicity in Deep Conversations: A Reddit Case Study
Vigneshwaran Shankaran, Rajesh Sharma
Preprint
Paper DataOnline social media has become increasingly popular in recent years due to its ease of access and ability to connect with others. One of social media's main draws is its anonymity, allowing users to share their thoughts and opinions without fear of judgment or retribution. This anonymity has also made social media prone to harmful content, which requires moderation to ensure responsible and productive use. Several methods using artificial intelligence have been employed to detect harmful content. However, conversation and contextual analysis of hate speech are still understudied. Most promising works only analyze a single text at a time rather than the conversation supporting it. In this work, we employ a tree-based approach to understand how users behave concerning toxicity in public conversation settings. To this end, we collect both the posts and the comment sections of the top 100 posts from 8 Reddit communities that allow profanity, totaling over 1 million responses. We find that toxic comments increase the likelihood of subsequent toxic comments being produced in online conversations. Our analysis also shows that immediate context plays a vital role in shaping a response rather than the original post. We also study the effect of consensual profanity and observe overlapping similarities with non-consensual profanity in terms of user behavior and patterns.
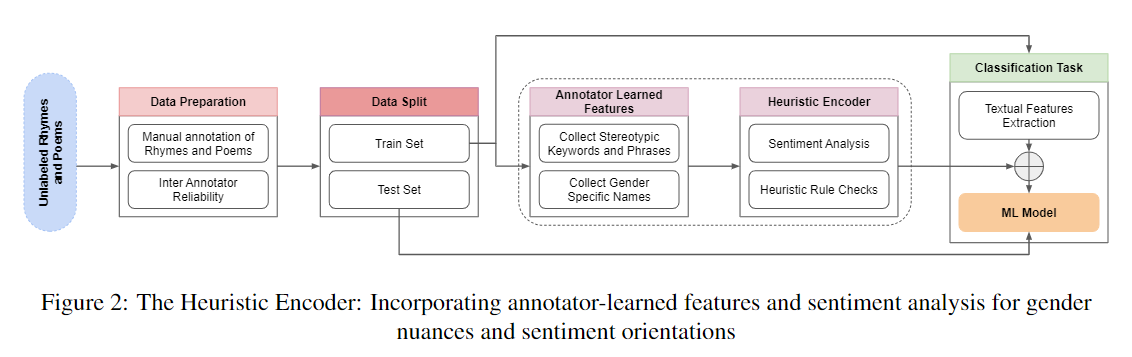
Revisiting The Classics: A Study on Identifying and Rectifying Gender Stereotypes in Rhymes and Poems
Aditya Narayan Sankaran*, Vigneshwaran Shankaran*, Sampath Lonka, Rajesh Sharma
To appear in LREC-COLING'24
Paper DataRhymes and poems are a powerful medium for transmitting cultural norms and societal roles. However, the pervasive existence of gender stereotypes in these works perpetuates biased perceptions and limits the scope of individuals' identities. Past works have shown that stereotyping and prejudice emerge in early childhood, and developmental research on causal mechanisms is critical for understanding and controlling stereotyping and prejudice. This work contributes by gathering a dataset of rhymes and poems to identify gender stereotypes. We then propose a model with 97\% accuracy to address gender bias. Gender stereotypes were rectified using a Large Language Model (LLM) and human educators along with a survey comparing their effectiveness. The findings highlight the pervasive nature of gender stereotypes in literary works and reveal the potential of LLMs in rectifying gender stereotypes and encourage further research in this area. This study raises awareness and promotes inclusivity within artistic expressions, making a significant contribution to the discourse on gender equality.
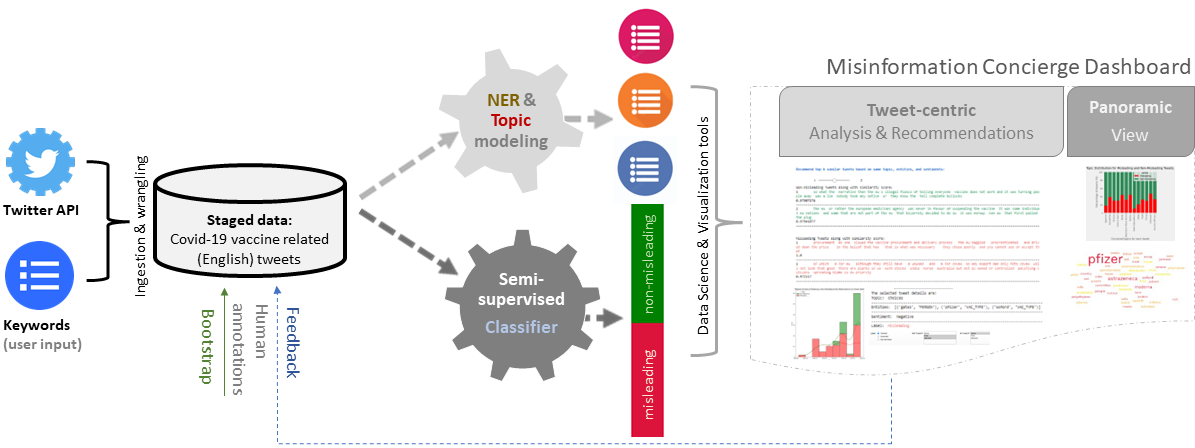
Misinformation Concierge: A proof-of-concept with curated Twitter dataset on COVID-19 vaccination
Shakshi Sharma, Anwittaman Datta, Vigneshwaran Shankaran, Rajesh Sharma
CIKM'23 Demo track
PaperWe demonstrate the Misinformation Concierge, a powerful tool that provides actionable intelligence on misinformation prevalent in social media. Specifically, it uses language processing and machine learning tools to identify subtopics of discourse and discerns non/misleading posts; presents statistical reports for policy-makers to understand the big picture of prevalent misinformation in a timely manner; and recommends rebuttal messages for specific pieces of misinformation, identified from within the corpus of data - providing means to intervene and counter misinformation promptly. The Misinformation Concierge proof-of-concept using a curated dataset is accessible at: https://demo-frontend-uy34.onrender.com/
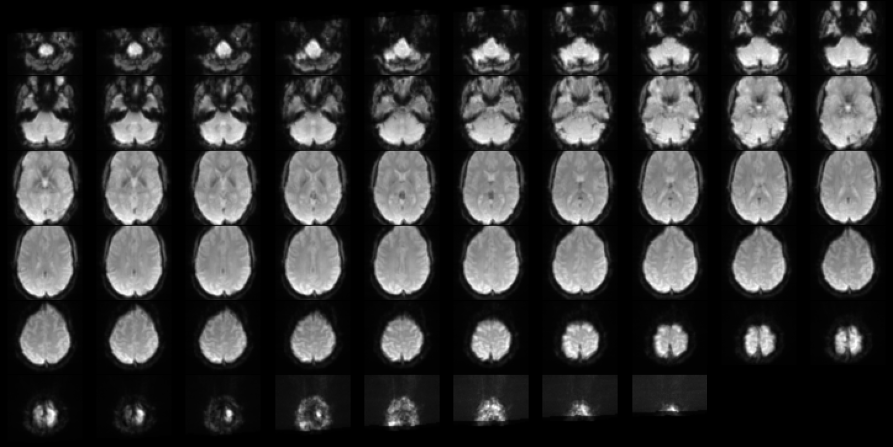
Multi-site Diagnostic Classification Of Schizophrenia Using 3D CNN On Aggregated Task-based fMRI Data
Vigneshwaran Shankaran, Bhaskaran V
Preprint
Paper CodeIn spite of years of research, the mechanisms that underlie the development of schizophrenia, as well as its relapse, symptomatology, and treatment, continue to be a mystery. The absence of appropriate analytic tools to deal with the variable and complicated nature of schizophrenia may be one of the factors that contribute to the development of this disorder. Deep learning is a subfield of artificial intelligence that was inspired by the nervous system. In recent years, deep learning has made it easier to model and analyse complicated, high-dimensional, and nonlinear systems. Research on schizophrenia is one of the many areas of study that has been revolutionised as a result of the outstanding accuracy that deep learning algorithms have demonstrated in classification and prediction tasks. Deep learning has the potential to become a powerful tool for understanding the mechanisms that are at the root of schizophrenia. In addition, a growing variety of techniques aimed at improving model interpretability and causal reasoning are contributing to this trend. Using multi-site fMRI data and a variety of deep learning approaches, this study seeks to identify different types of schizophrenia. Our proposed method of temporal aggregation of the 4D fMRI data outperforms existing work. In addition, this study aims to shed light on the strength of connections between various brain areas in schizophrenia individuals.
Miscellaneous
- Sub-Reviewer of the 2023 IEEE/ACM International Conference on Advances in Social Network Analysis and Mining.
- Master Thesis Supervision - Toxicity in Google Play Store Reviews: What, Where and Why? - 2022/3
- Co-organizer of the Computational Social Science(CSS) Workshop, Institute of Computer Science, University of Tartu 2022.
- Teaching Assistant - MTAT.03.319 Business Data Analytics, University of Tartu - Fall 22 & Spring 23. Lectures are available here (Access available to UT Students).